The Pivotal Role of Synthetic Data in the Era of Generative AI
A Transformative Approach to Training Data
5 minutes
6th of February, 2024
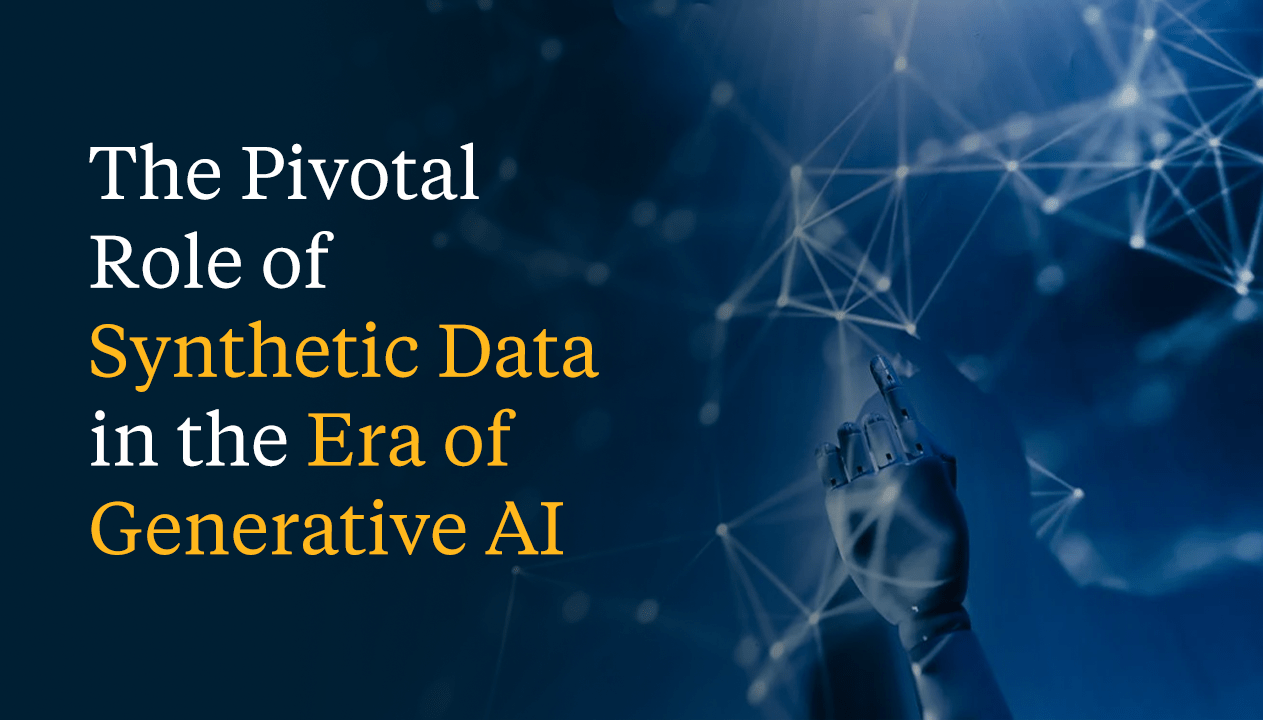
Centuries ago, Greek philosopher Heraclitus proclaimed, “Change is the only constant.” In today’s technologically dynamic era, we constantly strive to simplify complex processes, delegate mundane tasks to machines and bots, and reserve innovation and imagination for the human mind. In this evolving landscape, Generative AI emerges as a disruptor that is reshaping business and data interactions.
Refining crucial aspects of data labeling, annotation, and synthetic data generation is at the center of the Generative AI transformation . These elements are the keystones in powering AI to drive innovation across diverse industries, from automotive to healthcare.
Importance of Data Labeling and Annotation in AI
As AI applications grow, the demand for extensive, varied, and complex datasets has risen. Here, synthetic data generation steps in as a solution, especially in scenarios where real data is scarce or sensitive. Utilizing Generative AI allows for the generation of diverse datasets that mirror the complexity of real-world data while upholding privacy and compliance standards. This strategy is particularly pivotal in sectors like healthcare, finance, and autonomous vehicles, where data depth and quality can significantly sway outcomes.
However, as we embrace synthetic data generation, challenges loom. Surveys indicate most organizations use partially synthetic data, a blend of real and synthetic data. Text-based data is predominant, closely followed by tabular and image-based forms. It is important to recognize the challenges in fully synthetic data usage, particularly in ethical, secure, and sensible handling. Partnering with an enterprise that specializes in data mastery can help your organization navigate this complex landscape by filtering out data that is not just reliable but also pertinent.
Catalyzing Generative AI Adoption and Innovation
In this data-driven era, finding a transformative approach to data annotation, labeling, and synthetic data generation rises above service provision. Here are some ways to harness this power:
Lowering the barrier to entry:
Offering meticulously annotated and synthesized data empowers startups and smaller enterprises that are lacking extensive data curation resources to harness AI technologies. This fosters a more inclusive innovation ecosystem.
Enhancing model reliability and ethics:
The success of Generative AI models hinges on the quality of training data. Finding a solution that guarantees high-quality, diverse datasets, leads to more reliable and ethical AI models. This is especially crucial in sectors like healthcare, where AI decisions have profound implications.
Spurring industry-specific innovations:
Partnering with organizations that tailor solutions through data services that are fine-tuned to industry-specific needs empowers businesses to explore new AI-driven solutions that were previously unattainable due to data limitations. This approach opens doors for advanced applications in fields like personalized medicine and smart city planning.
Accelerating time-to-market:
Pre-built platforms and algorithms significantly reduce AI application development time. This rapid deployment is essential in industries where innovation speed is critical to success.
In the Generative AI areas, businesses will encounter a vast array of data types. Each of these comes with its own unique challenges and opportunities. From intricate medical images to complex autonomous vehicle sensor data, advanced algorithms can be tailored to manage, interpret, and optimize these diverse data forms, transforming them into actionable insights for various industries.
Encouraging sustainable AI practices:
The commitment to 'Green AI' focuses on computational efficiency and data optimization, contributing to more environmentally responsible AI development. This aligns with global sustainability goals.
Fostering a cycle of continuous improvement:
It is crucial to find a partner whose client engagement exceeds service delivery. At Akkodis, we establish feedback loops that encourage continuous AI model efinement, leading to a cycle of ongoing improvement and innovation.
Tackling data optimization challenges:
The escalating volume of data required for Generative AI poses challenges in data reduction and computational consumption. A twofold approach involves advanced techniques to distill data to its informative essence and a focus on computational efficiency, crucial for sustainable, cost-effective AI development.
Fueling innovation with Generative AI:
Looking to the future, the role of data in AI becomes increasingly paramount. Look for a data partner who continually refines their data labeling, annotation, and synthetic data generation. The Generative AI solution stack should not just be a set of tools; it should be a catalyst for innovation, enabling businesses to unlock AI's full potential.
Empowering Enterprises on Their AI Journey
Akkodis guides our business partners through the complexities of AI by equipping them with the right tools, strategies, and insights for success in this exciting era of Generative AI. Our offerings and frameworks are designed to be a long-term investment in ethical data handling, with the goal of elevating the quality of human life. To learn more about how Akkodis can help your organization in its AI journey, contact us today!